
Second, it is so far unknown how clinical parameters related to the amputation (for example, remaining forearm percentage, phantom limb sensation, use of prostheses 7, 13) and physiological phenomena (such as cortical reorganization) can affect the natural control capability of the prosthesis. 5)), which makes it difficult to obtain statistically relevant results. First, the described studies usually include too few subjects (according to our knowledge, up to 11 intact subjects and 6 amputees 12) and too few tasks (according to our knowledge, up to 12 (ref.

Often, non-invasive methods are based on the use of several electrodes to record sEMG, and pattern recognition algorithms to classify the movement that the subject is willing to perform, and recently, such a system has been clinically deployed ( Nevertheless, research in this field still suffers from a number of problems. 8), exceeding 90% in specific cases 2, 7. Excellent results have been obtained with invasive methods 9, while non-invasive studies usually show average classification accuracies of hand movements up to 80–90% (ref. Improvements over the conventional myoelectric control strategy have already been described in scientific literature 2– 11. Myoelectric prostheses could potentially improve the quality of life of hand amputees, but the control system hinders this advancement and it is one of the main causes of the low acceptance of such devices 1. Controlling the prosthesis is far from natural motion, and the user must undergo a long and complicated training procedure. However, the methods used to control such advanced hands are usually rudimentary, relying on sequential control strategies. In most cases the tasks that a prosthesis can perform are limited to opening and closing, but in recent years top-level commercial offers have appeared, including mechanically advanced prostheses that can perform several programmable movements. The work described in this paper aims to aid the progress in robotic hand prosthetics, making it possible to develop and test algorithms for movement recognition and force control on a scientific benchmark database.Ĭurrently, trans-radial amputated subjects (who are the majority of upper limb amputated people 1) can rely on myoelectric (controlled by electromyography, sEMG) prostheses. The application of these advancements of the field of prosthetics could greatly impact the quality of life of impaired people, but still faces many challenges.
#Matlab format not scientific portable#
The recent, rapid evolution of portable sensors and mechatronic technology has made robotics available in everyday life. The validation section verifies that the data are similar to data acquired in real-life conditions, and that recognition of different hand tasks by applying state-of-the-art signal features and machine-learning algorithms is possible. The database is targeted at studying the relationship between surface electromyography, hand kinematics and hand forces, with the final goal of developing non-invasive, naturally controlled, robotic hand prostheses.
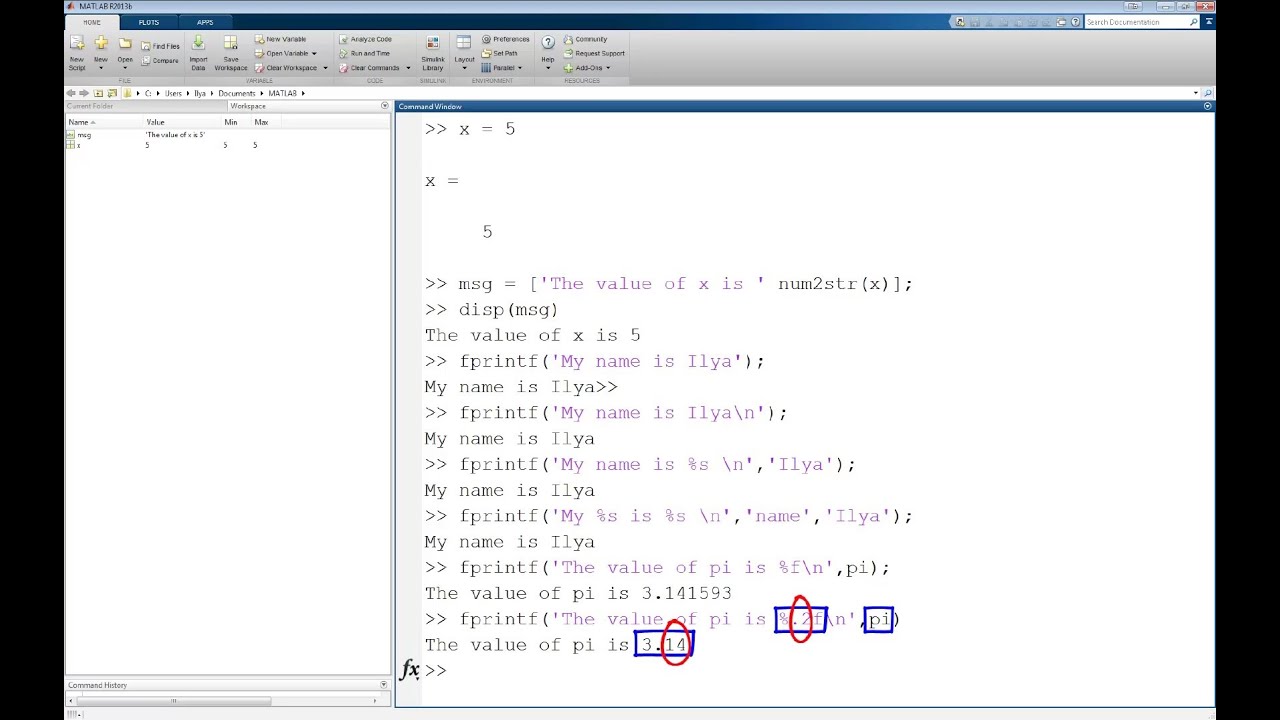
This work aims to close this gap by allowing worldwide research groups to develop and test movement recognition and force control algorithms on a benchmark scientific database. Meanwhile, scientific literature results are promising but they are still far from fulfilling real-life needs. The control of robotic prosthetic hands using non-invasive techniques is still a challenge in real life: myoelectric prostheses give limited control capabilities, the control is often unnatural and must be learned through long training times. Recent advances in rehabilitation robotics suggest that it may be possible for hand-amputated subjects to recover at least a significant part of the lost hand functionality.
